Artificial Intelligence
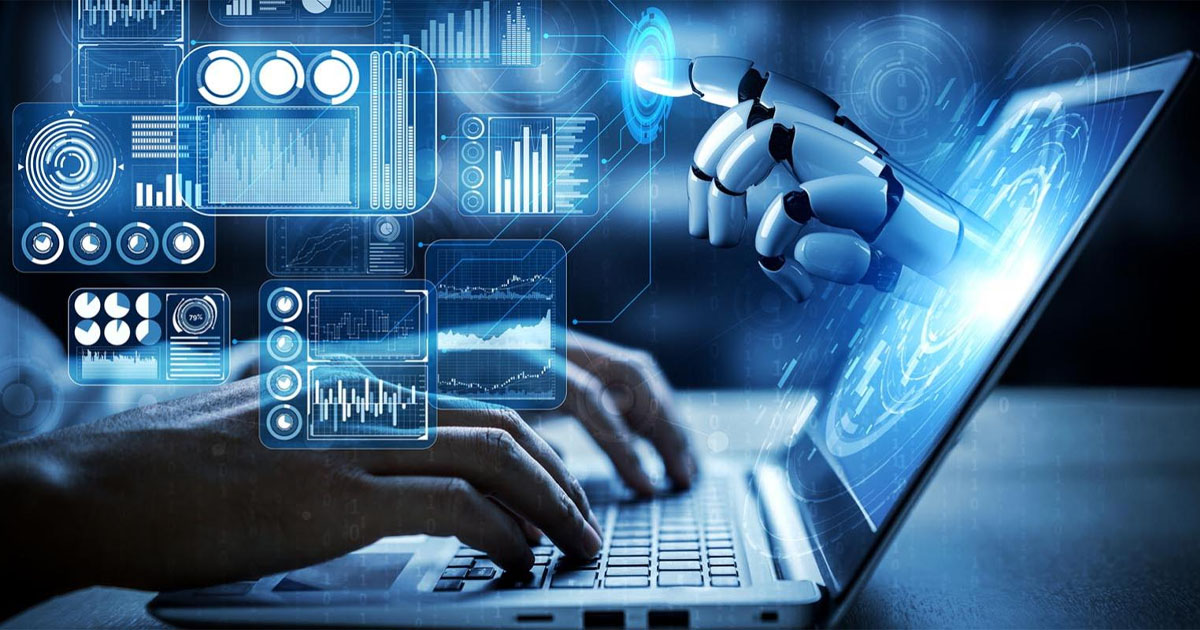
In this context, artificial intelligence (AI) refers to AI as a type of machine that is program to think like a human being to mimic their actions and think like humans to simulate human intelligence. The term “artificial intelligence” may also be applied to any machine that displays features associated with a human mind, such as the ability to learn and solve problems.
Artificial intelligence should have the ability to rationalize and take actions that have the most excellent chances of achieving the goal that it is trying to achieve. Artificial intelligence (AI) is a subset of machine learning (ML), which involves computers learning from and adapting to new data without human assistance. Through deep learning techniques, automatic learning is enable by absorbing much-unstructured data such as text, images, and videos.
Takeaways From The Event
A machine with artificial intelligence (AI) is a machine that simulates or approximates the brightness of a human by mimicking its behavior.
AI aims to enhance the learning ability, reasoning ability, and perception of humans with the assistance of computers.
AI is use in various industries today, from the financial sector to the healthcare sector.
The weak AI tends to be simple and oriented towards a particular task, whereas the strong AI can handle more complex, human-like tasks.
Several critics believe that the extensive use of advances in artificial intelligence can have adverse effects on society.
Knowledge representation:
With knowledge representation and knowledge engineering, artificial intelligence programs can make intelligent inferences about real-world facts and questions.
An ontology is a set of objects, relations, concepts, and properties that have been formally describing for software agents to be able to interpret them. Upper ontologies are the foundations for all other ontologies. As a mediator between domain ontologies that cover specific knowledge about specific knowledge domains (field of interest or area of concern), upper ontologies provide a foundation for all other ontologies. There is a need for accurate intelligence to have access to commonsense understanding, which is the set of facts that people are aware of. Ontology semantics are describe in a Web Ontology Language, which is the language use to describe ontologies.
In the AI research, tools have been develope to represent objects, properties, categories, and relations between objects; situations, events, states, and time; causes and effects; and knowledge about knowledge (what we know about what others know). It also includes default reasoning (assuming something is valid until it is told differently and will remain faithful even when other facts change). AI faces several challenges, including the breadth of commonsense knowledge (the average person knows atomic facts in abundance); and the sub-symbolic form of most commonsense understanding (many things need to represent verbally as facts or statements).
Formal knowledge representations are use in clinical decision support, scene interpretation, and knowledge discovery (finding “interesting” inferences from large databases).
How we learn:
An important aspect of artificial intelligence is machine learning (ML), which studies algorithms that improve automatically as they gain experience. An unsupervised learning algorithm finds patterns in a stream of inputs.
The two main supervised learning types are classification and reinforcement learning, and numerical regression. An algorithm uses variety to determine what category something belongs to – it sees examples from several classes and learns to classify new inputs. Regression aims to describe the relationship between inputs and outputs and predict how the results will change as the inputs change. It is possible to view classifiers and regression learners as function approximators; for example, a spam classifier is learning a function to map email text to one of two categories, “spam” or “not spam.”
A reinforcement learning agent is reward for good responses and punish for bad ones. It forms a strategy for operating in its problem space by classifying its reactions. The transfer of knowledge from one problem to another is called transfer learning. By computing complexity, sample complexity (how much data is needed), or other notions of optimization, computational learning theory can assess learners.
What you need:
 Search engine optimization
Using AI to search through data intelligently is one way. Which AI can solve many problems with numerous possible solutions. Using search engines can reduce the amount of reasoning required. The logic of logical proof can be view as an inference rule that leads from premises to conclusions. The means-ends analysis is a method of finding out how to reach a goal by examining all the existing subgoals and plans. As part of the robotics process, local searches are use in configuration space when moving limbs or grasping objects.
More than exhaustive searches require a rapidly growing search space (number of places to search) for most real-world problems. The result is a slow or never-ending search. To solve many situations, using “heuristics” to prioritize choices based on their likelihood of achieving a goal is helpful. Heuristics can also help eliminate some options unlikely to lead to a plan (called “pruning the search tree”). Heuristics provide the program with a “best guess” for the solution. Searching for answers is limite heuristics.
Random optimization, beam search, and simulated annealing are related optimization algorithms. Evolutionary computation makes use of optimization search as a tool. They may start with a population (the guesses) and then let the organisms. Mutate and recombine, selecting only the fittest every generation (refining the guesses). Gene expression programming, genetic programming, and genetic algorithms are evolutionary algorithms. A swarm intelligence algorithm coordinates distributed search. Popular swarm algorithms are particle swarm optimization (inspired bird flocking) and ant colony optimization (inspired ant trails).
Lights that smart:
Carnegie Mellon developed intelligent traffic lights in 2009. Since then, Professor Smith has started Surtrac, which has installed intelligent traffic control systems in 22 cities. The cost is about $20,000 per intersection. In the corners where it has install, drive time has reduced by 25% and traffic jam waiting time 40%.